Recently Professor Gina Brown gave a talk to the Worshipful Company of Information Technology about the use of state-of-the-art Artificial Intelligence (A.I.) in Gastrointestinal Imaging.
Red Trouser Day founders Paul Finch and Julia George organised the lecture to raise awareness amongst the WCIT members.
You can watch the full lecture by clicking on the video on this page.
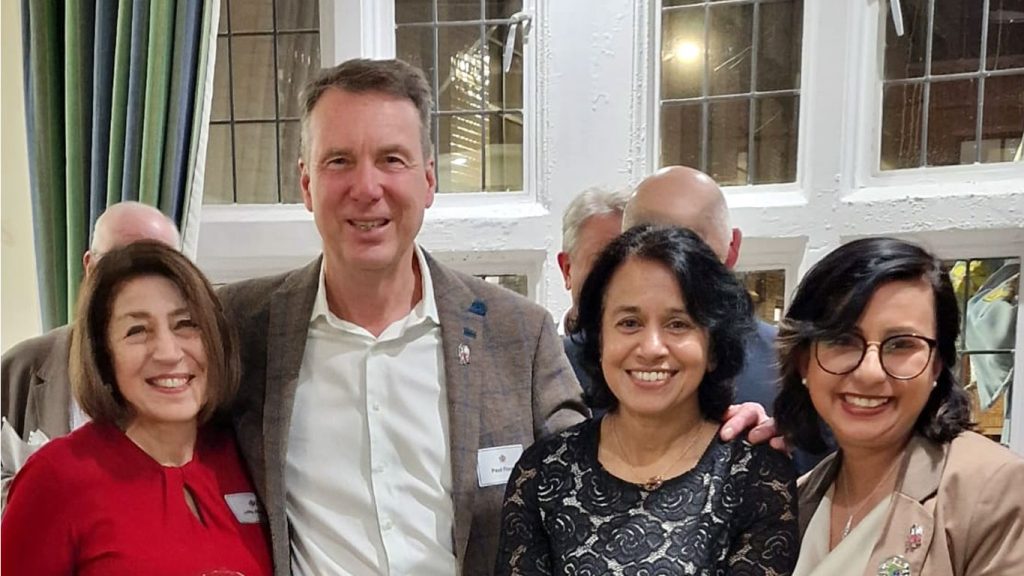
From left to right: Julia George, Paul Finch, Prof. Gina Brown, RTD ambassador Rosey Woodhead
Transcript
[Music] I think you’re all familiar with the headlines that we um are seeing pretty much every week um and it’s all to do with Workforce shortages and in Radiology specifically um what we’ve had is over the years an increase in technology but not necessarily commenor increase in the workforce to deal with it all and it’s great for patients because you know you could go into a hospital now and instead of just having an x-ray and be sent home you’re probably going to have at least half of those tests but it’s not so great for the people on the sitting behind the the workstations going oh this patient I now got to report one two 3 four five tests and then I’ve got to look at it again in about three months time to see how it’s changed and there’s about you know regularly in our works state we’ve got about a 100 Radiologists now we previously had when we first started in Imperial years and years ago it might have been about 15 so you know you can see what’s what’s happening but we still can’t keep up so that’s our challenge um the other challenge is it doesn’t happen overnight um I started medical school I was going to reveal my age um in um 1983 spent five years as a medical stud student I spent longer because they didn’t have the foundation years in in that time so I spent three or four years as a medical registar doing diabetes respiratory medicine thir the I did my MRCP and having got all that they let me in to do Radiology training because we need a solid background in medicine before you start interpreting disease and relaying that information knowledgeably to your clinical colleagues because otherwise you become a kind of instrument rather than an opinion and I always say to my colleagues we are clinical Radiologists we are not technicians we are doctors first and we’re using the Imaging to help patients get the right treatment get the right diagnosis so after after doing those years of training doing the exams you then spend a bit of time doing sub special training and in my case I spent time doing research uh which was um a higher degree um and then eventually became a consultant and that’s where the trouble begins but of course um we would love this wouldn’t we just you know I could sit down have a large cup of coffee and let the machine do it all but it’s not happening it’s not happening right now maybe in in decades to come it might but for the moment we are using it uh AI we are using it right now in most hospitals uh the Machinery will allow you to um automate segments and and measure nodules in the lungs all of that sort of thing that’s that’s that’s that’s here and now we’re using that but uh there are there’s more that can be done and I I’ve just sort of taken this scraped it off the internet but it’s a pretty good summary of what what we’re likely to be able to do so this is just large language modeles in imaging you can you can put them through um what essentially is database analysis to come up with usable prepopulated clinical documents so instead of the the clinician taking your history you know line by line by line and spending half an hour writing they can be listening to you and populating what’s needed that’s unique to you from the clinical documentation that they that they’re finding out so so there are there are things that we can do to improve workflow of course interpretation of course we can have prompts you know you’re looking at a scan and you know it’s a particular condition of course you want to to know what what are my what are my mental checklist what I should what should I be looking for in this patient with this condition so if they’ve got repal cancer have I looked for this have I looked for that so th those are the sort of things that are going to improve not just the workflow but also our accuracy you can feed this information in to an algorithm that’s taken the the knowledge and evidence base and produce treatment options of course all of those things can happen so we we’re already there but it has to be integrated better and in a smarter fashion for us to use it and I think what what’s missing is probably a really big dialogue with the people that need it and that are using it so the Medics and getting them engaged is is one of the challenges because they’re sort of it’s a catch 22 they’re way they’re they’re in a clinic there’s 40 patients waiting to be seen by midday they’re not going to spend half an hour talking about it and that’s the problem but so we need to have some bandwidth to to spend some time when I first started in Radiology a long time ago we would have an afternoon of development time it was just built into our um our rotors not every week once every three to four months and we would spend that time looking at our machinery and optimizing the protocols that afternoon saved multiple afternoons in terms of throughput just investing a bit of time um we’ve over the last 15 20 years our work plans our job plans and this is why I I switched to the university used to be seven clinical sessions seven direct patient clinical sessions through three sessions of thinking time research audit writing teaching others thinking about how you can improve your protocols that’s been gone so we’re all on on the NHS side we’re all on nine and one so we’re working nine sessions of direct clinical care and one session which is four hours to spend on yes I know recouping I think so um that I think is where we’re at so I suppose one of the one of the solutions is to give up give the r give the Radiologists give the Medics some bandwidth to start thinking about this stuff again because that is really what’s needed if we to improve things nonetheless there’s some exciting discoveries there’s some exciting things going on um the UK has led research in in uh improving the diagnostic uh capabilities of scanning and there is technology that will fuse say a CT scan or an MR scan which is what we’ve got there to an ultrasound that’s a prostate cancer the ultrasound doesn’t show where the cancer is but if you overlay the scan onto it then um the technologist or the doctor whoever’s doing the biopsy can direct themselves accordingly and get a proper sample and it’s not just a sort of shot in the dark so so these are the sort of things that we’re using right now and it and it’s working well th this again is a is a a case use that is on our scanners right now so if we if we put a line on on a metastasis it will automatically identify outline it measure it and then we can assess the the spread of that metastasis over sequential scat it’s not perfect but in in the majority of cases it’s good enough but what should we be careful about in uh AI in medicine the first one um I think you’ll you’ll see that this was the the first um image I showed where where we were using AI to um outline nodules but there is this thing called radiomix uh which uses the same Principle as genomic in the sense that it’s looking at all sorts of things that we can’t perceive with our eyes so it’ll be looking at the original source code the original um not source code the original um data in the image to have look to look at say uh they’ve got various coefficients uh int voxal coherence there’s all sorts of what I call mumbo jumbo but actually produces M multiple sets of data like this and lots of Curves and lots of um things that you can then you know produce a map of the characteristics that are seen within a single Pixel of an image and presume that that may have some hidden aspect about the tumor the problem with that is what we’ve observed in radiox is there’s lots of activity in this area it’s very easy to do you can put regions of Interest over something generate data produce a signature and the problem and this is just the the huge increase in rmx papers over the years the problem is you get this nothing actually works in practice because you’re overfitting the data you’ve got so many data points of course you’re going to find a pattern you’re going to but you’re not going to be able to replicate that in another person or another machine and so to me when somebody tells me uh about radiomic I am highly skeptical in the same way that I’m skeptical at genomics when there’s no hypothesis there’s no understanding of the mechanism and it’s a fishing Expedition oh let’s see what what the signature throws up and that’s what you end up with absolutely overlapping things so what’s the other thing that we should be careful about well this is a a paper from the lanet um just published very recently about using AI in breast cancer screening which on the face of it seems like a really logical um approach what they’ve discovered was the current practice in breast cancer screening is that you have radiologist one who looks at everything radiologist two that looks at the same panel of mammograms and if they agree that it’s normal they agree it’s normal and and it gets passed off as normal and if one or other disagree there’s a there’s a consensus meeting to discuss why one thought it was normal and one didn’t and if both agree that they were abnormal the patient is recalled the rates when you’re in screening the rates of picking up cancer are vanishingly small they’re not the same as when somebody comes with a lump that they present with it’s about seven per thousand so you have to be certain that the screening program is fit for purpose in that you’re picking up those seven out of a thousand and you’re not missing a proportion what happened with the AI machine is that if you left the AI machine to its own devices it would pick up three quarters of what a trained radiologist could do and missed 25% so that’s a nonstarter and it also identified a whole bunch of Legions that the radiologist would have dismissed but in amongst that bunch of Legions were some cases that that went on to become interval cancers so nothing is 100% on either side so what do you do with that information well you do a cond a well conducted study and model the different scenarios so in one scenario they used the AI as the second reader so that if this if the AI found something that the first reader which is a human didn’t notice there would then be a discussion about that with the third radi with not the third the second human so there’s three processes going on here that actually did save 40% of the radiologist work over time so what happened then was that the second radiologist is freed up to the more mammograms um so but that means that they then are only limiting to the that 40 40% so 60% ends up being double red um as opposed to the 100% so it’s saving about half the time so th those are the sorts of things that um if we think about it we can we can use but to think that the Imaging um can be automated to that degree is is perhaps a little bit optimistic I’m sure sure that with more and more data and more and more validation they will get better so 15 cm 15 mm lesion is a sort of strength of accuracy something smaller than that the machine’s going to miss in all probability and what we can do is teach the machine the things that it’s missed and gradually it will it will start to improve but it’s it’s not a bad shot isn’t it for for a first um for one of the I think it’s the second publication in this region uh in this topic and it will definitely improve so you take those principles um but I think everyone knows in it and it’s it’s a well it’s wellknown truth rubbish in equals rubbish out so data input is so important um making sure that your ground truth is accurate is quite uh key and um I’ll I’ll sort of talk about some of that now in in some examples of my own um uh research um because that’s that’s where I think it becomes quite interesting because we have different ground truths so if you had a 100 Radiologists in a room and you asked them about this lymph node you would get any one of those six answers that is a problem because if one of those Radiologists is feeding the data into the algorithm to train um machines as it were it’s going to be not right or it won’t be in agreement with somebody else um the the reality is and I I’ll talk about lymph noes in a moment but that’s just an illustration of of of ground truth so in fact lymph nodes are a fascinating area for me certainly and I think hopefully for for for everyone around to understand what their role is in in cancer um long long ago in 1932 um a pathologist called CET Jukes he wasn’t so far away from here was in St Mark’s Hospital which was in um East London for for a time in in in linked to bonuse U not so long ago he um showed that if lymph nodes were seen in a surgical specimen the chances of the patient surviving was only 7% so shocking number 93% were dead within 3 years but this was in 1932 and and surgery was literally done with a sort of pull and snatch approach even by the great St Mark surgeons it was not the surgery we see today and yet because of this work and because of the work subsequently lymph nodes has been a big thing if anyone’s ever um encountered oncologists the first thing I’m talking about is lymph noes lymph noes um and the problem with lymph nodes is that we see lots of lymph nodes and some of them can be benign and some of them can be malignant and they get big or they can be small and you know we there is no distinct redeeming character of a lymph node that tells us one way or the other but what was interesting is that when the radiologist is saying the lymph noes are enlarged there is a positive Improvement in the patient’s survival which is completely counterintuitive to what Dukes was saying and we’re the research that I’m doing now is is getting our heads around that and also assisting Radiologists in learning to report lymph noes in a totally different way in other words if they’re multiple and enlarge it’s a good prognostic Factor but actually it’s useful to count them you know if a patient’s got a Cancer and they produced a lot of lymph nodes that’s actually a good prognostic factor and how many they produce is likely and that’s what we’re testing in our research is likely to be something that’s a marker for a very strong patient driven immune response as a healthy immune response so blasting those lymph noes with radiotherapy is going to have the opposite effect to what you want you want to augment that lym mode response and I think now we’re you know as we’re entering this uh next era of research in drugs we’re realizing that actually the drugs that we’re using that to just clobber and you know diminish the tumors may be the wrong way around it may be better to start to develop drugs that augment a good immune process if that patient’s already producing a good immune process why don’t we augment it so so that that’s the sort of um way that things are going so the Pathologists are now their their heads are turning as well because if you have lots of enlarged lymph nodes it’s a good prognostic Factor so that was a publication from the pathology group in Germany and and why is that why should that be and the reason is that tumor within a lymph node is presenting an antigen the antigen is viewed as hostile by the host immune system the more antigens the tumor produces the more likely it is that the patients going to recognize them as foreign bodies and Goble above and deal with them the problem with some tumors is they don’t produce antigens they hide then there’s no lymphatic response and there is an absence of lymph nodes so what happens then to patients how do they what’s happening there that we need to understand well the secret is in this image here which is showing something that um we discovered on Imaging and was rather neglected by pathologist as a so what um but actually it is the most important prognostic factor in a patient with col or rectal cancer so this is the phenomenon of tumor growing into a vein and forming these which are not the same as lymph noes they’re very different they’re kind of you can see the shape of them they’re um spiky they’re following a vessel they’re turning Corners this is extramural Venus Invasion and we see it in about just under half of the patients you present to us with repal cancer and with and when you see that about half of those patients develop liver metastases or lung metastases if you turn it the other way around if you look at the original pathology of patients who have had Li metastases in 95% there has been vascular invasion in the primary tumor whereas in the lymph nodes it’s a sort 50/50 so so what we have observed is that firstly Venus invasion is common secondly it is very easily recognized and much more easily recognized on the images than it is on the pathology the reason being is that we see the anatomy in Via we see the veins we see them as very character istic structures so what happens is that when we see something like that we know that that is a marker for the spread of tumor to the liver and if we can block that if we can understand why that happens and if we can prevent that from happening in patients with tumor or at least prevents that tumor from getting into the veins in the first place then we will be reducing the cause of cancer death in patients with bound cancer so how should we report this I think maybe perhaps people in the room can now recognize that there’s a there’s a lump there and then behind it are two sort of stringy bits what are they they’re vessels so that’s a tumor lump growing and interrupting a vessel that’s how easy it is it shouldn’t be beyond the ability of machine learning to recognize tube lump tube it shouldn’t be so that’s that’s our next step is to start to get that information sort of um recognized um for Radiologists so that they can be alerted to the possibility that this patient has a poor prognostic risk factor it cannot be a lymph node the no nodule is traing along a vessel so so by understanding the pathogenesis we avoid the rubbish in rubbish out we actually look at something that’s meaningful that we know is prognostically important for the patients chances of surviving their disease and we can start to map it and look for it on the scans that we see the fact that it’s being neglected by Pathologists means that some of the existing data sets are not readily usable at the moment so we have to go back and start looking at them again which is a task that I’m about to embark on so the other bit of evidence is that the or origins of lymph nodes and distant metastases are not the same so so the sorry the T the tumor within a lymph node you can do its clonal um uh makeup you can do its profile bit like a family tree and if uh the tumor in the lymph node has gone to the liver then the family tree should be the same it should have the same characteristics but what we find when we look at things is that the lymph nodes have a totally different profile to the distant metastasis so in other words tumor is bypassing the lymph noes to get to the liver metastasis so in in that situation we are also seeing that the tumor is evading the thing that’s going to fight it it’s evading the lymphatic system how do we make those tumors visible to our first line of defense the immune system that’s the that’s the question and that’s what my research is going to be addressing so how are we going to do that first we’ve got to identify the tumors we’ve got to find find the tumors with these tumor deposits which we can do on Imaging much more readily than the Pathologists the next thing we do is obviously we’ve confirmed that the tumor deposits are associated with much worse survival than lymph nodes lymph nodes good t because it’s bad um and the next thing is to understand the what’s going on in the biology around those areas of vascular Invasion how is it that that tumor has successfully invaded into a vein and is now on its way to other structures there’s a battle going on what we call the seed and soil and that’s that’s the hypothesis that I’m developing and working on with um colleagues at uh the beats and lab um at leads and at uh niman in in the Netherlands and what we going to want to do is to look at the immune makeup around vascular Invasion around the advancing border of the tumor to see and we think this is going to be the thing that there will be a lack of the tumor infiltrating lymphocytes the the ones that are supposed to battle against the tumor so all the all the things colored in blue are the things that the patient is doing to stop tumor spread and all the things in red that I’ve cartooned here are the things that are promoting cancer spread what we want to do in the future is get get more blue and less red and the way to do that is to um identify the things that improve the patients chances of fighting that vascular Invasion because our hope is that because only 50% of patients with vascular Invasion get liet there must be something going on in the 50% that doesn’t that stops them getting it what is that and what can we do for the patients who haven’t got that thing and it’s probably IM related but that’s what we need to find out because there’ll be there’ll be a Cascade behind that that’s driving that process and that’s what we need to study and we have to study it uniquely in those tumors where there’s emvi visible on Imaging so that we can use that to prognosticate right at the beginning and get the patients on the correct treatment because what we’re doing isn’t working so um the the trials that we’re doing to look at this uh with driven is the big one is our Flagship trial we’re hoping to to get funding for a thousand patient Imaging pathology study this will serve two per well multiple purposes firstly nothing has been established like that before there’s no there’s no library of Imaging lined up against pathology side by side the histology sections that we’ll be able to get from that will be able to be looked at in much more detail we’ll be able to do immune staining immunofluorescent staining we’ll be able to look at the proteins and the genes around the tumor that has been responsible for this kind of devilish spread um another study we’re doing is looking at Patients who’ve developed recurrence to find out if as we believe that the roote for that recurrence has been through the Bas the pathway and again to study the original tissue to see how we could have prevented those recurrences we’re doing a a patient-based um study of intensifying surveillance for patients who unfortunately have vascular invasion in their primary tumor to Pro to to enable earlier detection of that spread and earlier treatment and then finally we’re mapping those tumor deposits on the um sections on the images and handing them over over to the pathologist so that when they’re looking at the specimen they see what we’ve seen and Sample what we’ve sample so that they’re accurac improves because currently they’re in picking up 10% of patients with tumor deposits whereas we’re picking up 40% so that that’s why um then that needs to improve so um that’s the sort of um thing that I hope to do we’re we’re sort of looking for funding to get some pilot work done but some of the things that we want to do is look at some samples like that where we match them and look at the immune um profiling the um what we call the the chain of events that leads to metastasis so that so that’s what we’re going to be able to do and and the thing is is that if you can feed that in to um a data set you will be able to produce a a unique profile for patients that based not just on their Imaging but also on their immune landscape just from a biopsy and integrate all of that to a really much better refined um prognostic staging system that the patient will be able to get from the biopsy um so the staging the biopsy the um uh post treatment staging all of those things will be able to really properly what we mean by properly personalizing treatment and not not words that these are how we’re going to get there so um the these are sort of exciting things that we’ve noticed um just in some very very pre pre-preliminary work which is that the the vascular Invasion uh tumors are are immune cold there’s hardly any um fighting immune cells in and around them compared with the um nonvascular Invasion tumors so there is something going on here that we need to get on top of um the other thing we’re going to do is um and this is what we’re doing with St Marks is mapping the lymph nodes uh and Counting them and that is so painful to do a radiologist going through each scan putting a little circle around every single lymph Noe that we are in the midst of um getting a AI um uh machine learning um program using nnet um type software that is going to be um a great tool we think because if you can say to a patient automatically you know as soon as they’ve had the scam you’ve got 40 lph noes you know that’s an amazing um um bit of information that you can give to Patient say well you know your immune response is is doing you a favor do what keep doing whatever you’re doing because your your that that bit of you is is working well so um yeah that’s that’s what we do we sort of measuring out these limites another study we’re doing which is in a totally different um area which is patient patients currently are having a lot of O overt treatment they’re being put through the mill you know radiotherapy surgery colostomy bags and and actually a lot of the patients that we’re seeing thanks to screening are early stage can we identify them and if we can do so can we prevent them from having major surgery and just have a local excision instead so that that’s the uh other aspect of of what we’re doing and what we’ve done is we’ve created a reporting tool again it’s going to need eventually large language model um processing to to produce something that will enable first the machines to link up with the images but also with a with a final report something that could easily be done because this is we’re looking not at the vessels and the veins we’re looking at the tumor simple question is there enough muscularis is there enough rectal wall preserved around it for a local exision to be performed so th that’s what we’re going to do um we’re going to um increase that little green wedge which is the only patients now with early rectal cancer that get a local exision to a whole green and that’s what we want to do so that patients really they if they’re going to go to the trouble of putting themselves through screening they benefit from having been screened to find an early cancer and not be put through some awful torment and then I think it will encourage patients to come forward because they’ll know that there’s a benefit um so there we have um the preserved trial and that I think hopefully this is a step wedge uk-wide um Custer randomized trial and we’re hoping that within a year or two this will become a common place um but but the other thing is we’re doing a lot of patient questionnaires and um hopefully we can use this tool to improve and streamline the health service for early rectal cancer so um that’s that’s our sort of hopes um so I’ll just move to the there’s so much that we can do at each step of the pathway of a patient having um rectal cancer from diagnosis to treatment and and these are the things that we we hope to do so generative language models reporting tools workflow modeling systems um machine learning to Aid diagnosis is not going to replace the radiologist but free up their time to do more things um and large data sets to help us with some predictive modeling modeling for individualized uh risk scores these are things that I think will work and we’ll we’ll be able to do so within you know within five years really there’s no reason why not if if we could get a little bit of a leg up with some pilot data but these um I just want to sort of acknowledge all the the fellows the research fellows um who are busy beavering away all of these projects and our trial team um and then all the hospitals that are taking art around the country um and that’s just the the start of things but um thank you very [Music] much